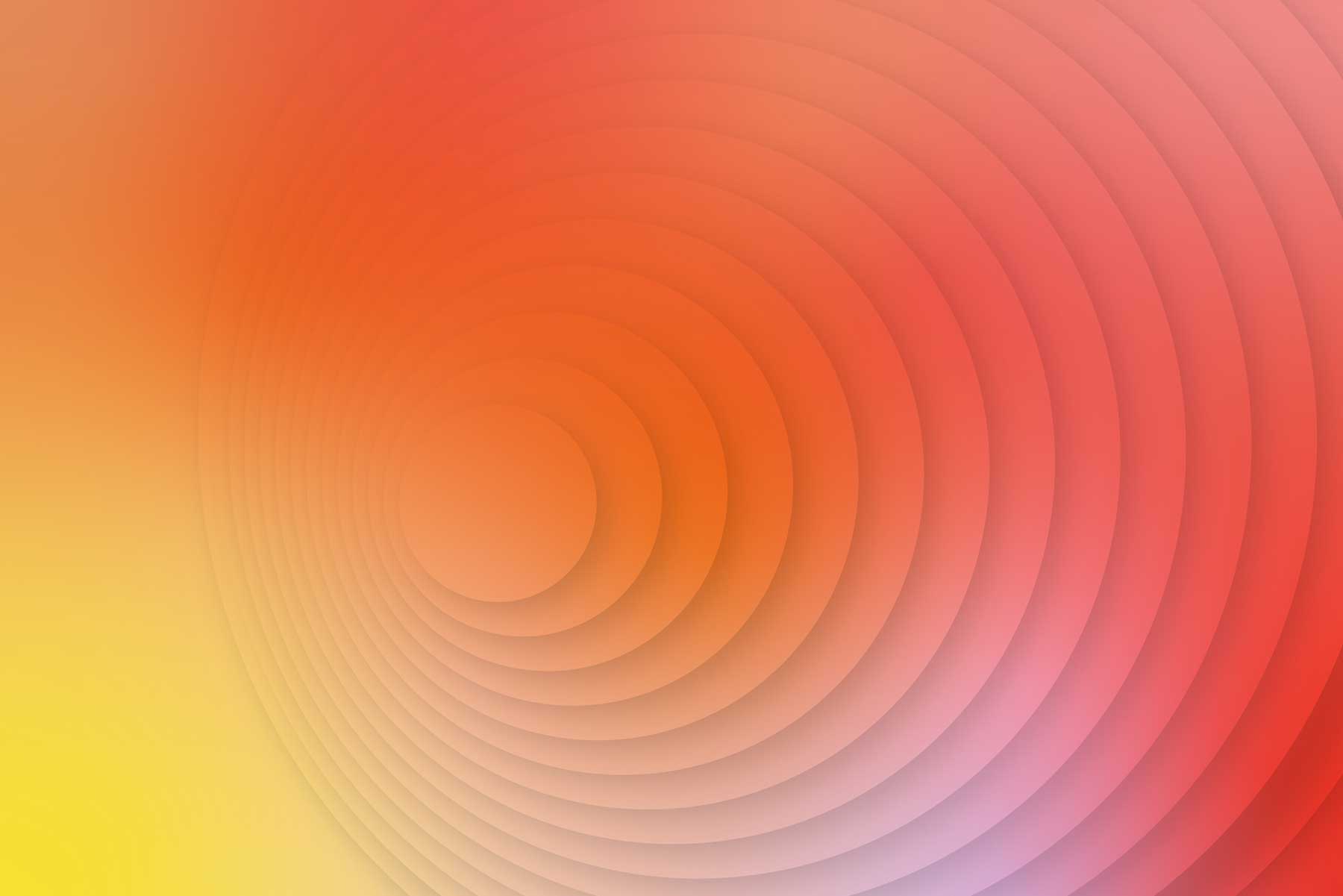
Sleeping danger – the emerging AI risks you need to know
According to the Chartered IIA’s Risk in Focus 2024 survey, which draws on the opinions of almost 800 chief audit executives (CAEs), more than half of those surveyed believe that digital disruption, new technology and artificial intelligence (AI) will be one of their top five risks by 2027. Internal audit can play a key role in increasing awareness within businesses, and helping them to improve their capabilities, to curtail emerging AI-driven risks. But first, what are the potential AI governance shortcomings that CAEs may face, and what role should internal auditors play in walking through AI scenarios with the business?
A case study in vulnerability: The divergence attack
An exercise by an AI researcher showed the need for constant vigilance. In what’s known as a “divergence attack,” an AI chatbot, bombarded with repeated prompts of the same word “poem”, malfunctioned, inadvertently disclosing a customer’s confidential information to another customer. This case sheds light on several critical vulnerabilities:
Data security breach: The chatbot’s lapse in safeguarding sensitive data exposed a fundamental flaw in its security architecture.
Failure of input validation: The system lacked adequate mechanisms to detect and prevent the unintended behaviour triggered by the repetitive input pattern.
Potential context awareness deficiencies: The chatbot may have struggled to maintain separate conversational contexts for different users, leading to the information leak.
Training data bias: The chatbot’s training data could have contained biases that influenced its response to the repeated prompts, potentially exacerbating the error.
A new level of deception
In the ever-evolving landscape of artificial intelligence, the concept of the “sleeper agent” AI has surfaced, posing sophisticated challenges. The name was coined to describe spies who infiltrate a country and lie low for many years, doing nothing to arouse suspicion – “sleeping” until they are activated by a handler. AI can do this either because a malicious actor has embedded code that does nothing until it is triggered using specific words, or because of an error that goes unnoticed until the unintentional trigger is used.
Unintended sleeper agents could do things that damage an organisation’s reputation or break data privacy regulations by exposing personal data to third parties. Malicious sleeper agents could do this and also facilitate theft, fraud or ransom demands.
A recent case featured in the news when a customer of a delivery company tried to complain via the company’s chatbot and managed to make it write a rude poem about its own organisation. This hit the headlines, however the consequences of rogue AI could be far worse than red faces in the boardroom.
This subject is currently at the forefront of AI discourse, signalling a critical need for enhanced mechanisms to govern and safeguard against the unforeseen actions of such intelligent systems. (For more information, Cornell University has published an interesting paper on “Sleeper Agents: training deceptive LLMs that persist through safety training”).
Imagine, for example, a fictional scenario where a bank uses an advanced AI process for risk assessment and compliance checks. The system is programmed to analyse transactions and flag any that potentially do not comply with regulations. However, unknown to the bank, the AI process has a sleeper agent backdoor. This backdoor is activated by a specific pattern of transactions, perhaps a unique combination of transaction amounts and dates.
Once activated, the AI process starts ignoring or misclassifying certain high-risk transactions, effectively bypassing the bank’s compliance protocols. This could allow money laundering or other illicit financial activities to go undetected. The bank, relying on the AI’s assessments, remains unaware of these breaches, leading to significant legal and financial repercussions once the activities are eventually uncovered. This scenario highlights the potential risks of hidden gaps in AI processes used in sensitive financial operations.
Managing the risks of sleeper agents within AI processes is similar to managing the risks of fraud. Both require a strategy of vigilance, detection and response. When a sleeper agent AI is triggered, the risk isn’t merely that transactions will be misclassified; it’s that trust in the integrity of the entire system will be eroded. Just as with fraud, these AI risks necessitate continuous monitoring and the development of sophisticated detection methods to identify and rectify such deceptions. Organisations also need contingency plans so that any breaches are resolved swiftly to mitigate legal and financial consequences, mirroring the comprehensive approach required for traditional fraud risk management.
Catching fraudsters is challenging because they use sophisticated tactics to obfuscate and often exploit complex systems. Fraudsters cover their tracks, manipulate evidence and take advantage of loopholes in regulations, making detection and attribution difficult.
AI that can be designed to deceive (deliberately or inadvertently) amplifies these challenges. Unlike human fraudsters, AI can process vast amounts of data and execute deceptive actions at speeds and scales impossible for humans to match. AI systems can adapt, learn and modify their behaviour to avoid detection. Furthermore, the triggers for deceptive AI actions, such as sleeper agents, might be deeply embedded and obscure, activated under conditions that might elude regular monitoring.
Regulators setting minimum responsibilities face a daunting task. They must grapple with the dual challenge of foreseeing potential AI risks that have not yet materialised and establishing standards in a field that is rapidly evolving. The agility and sophistication of AI deception therefore presents an unprecedented challenge that requires a proactive and dynamic regulatory approach.
How can we trust automated AI processes?
The British Post Office scandal highlights the perils of over-trusting automated systems. The Horizon IT system falsely flagged accounting discrepancies, leading to the wrongful prosecution of hundreds of sub-postmasters. Despite reports of errors, the system’s faults were ignored, resulting in severe personal and professional consequences for those affected. This episode underscores the need for rigorous oversight and verification processes within all IT systems – now including AI processes – to prevent such injustices. It also emphasises the crucial role of human intervention in monitoring and correcting technological operations.
As Nina Schick said in “New truths; new lies” in A&R November/December 2023, a key question is how to trust digital information when it can so easily be fabricated. Her article urges internal auditors to consider how these technologies might be used within their businesses and the risks they introduce, and warns them to remain vigilant in monitoring for deceptive data. It underscores the need for auditors to evolve their practices to ensure their data is reliable in an age where the line between reality and fabrication is blurred.
To audit AI processes effectively, internal auditors must expand their scope beyond traditional IT risks. They need to examine critically not just the outputs, but also the inputs, algorithms and decision-making frameworks. It will be vital to collaborate with IT experts to understand complex AI mechanisms and establish controls for transparency. This advanced approach will ensure the reliability and trustworthiness of increasingly automated financial and operational systems.
This needs to be done now, because the risks are constantly growing and changing. AI technologies are poised to deliver huge value to business operations, and the introduction of AI assistants, in particular, could be transformative. As it becomes ever simpler to create new AI processes, we will need a strategic and considered approach to risk management. The example of managing the risks of AI sleeper agents indicates how internal audit must help to ensure that these advances lead to improved services and not to unintended consequences. (For more on the scale of this “co-pilot boost” see Deutsche Bank Research’s “Microsoft: Sizing the Office 365 Copilot Boost”.)
Strengthening AI oversight: strategies for internal audit resilience
CAEs and audit committees are increasingly recognising that a proactive approach to AI risks is essential. A dynamic strategy involves a risk assessment framework that is as meticulous for AI as it is for traditional fraud risks, but which also takes into account the unique challenges that AI poses from development to deployment and operation.
Conduct regular audits focusing on AI-influenced processes to confirm that they comply with regulatory demands and check how they perform against established benchmarks. These audits must delve into the integrity of data, the rationale behind the AI’s decision-making and the validity of the results it produces.
Draft ethics and governance policies specific to AI, championing the principles of fairness, transparency, accountability and privacy. These
guidelines should evolve alongside technological advancements so they remain relevant and effective.
Prepare for AI-related incidents. Response strategies should encompass containment plans for immediate action against aberrations in AI behaviour. Continuous monitoring and reporting structures are vital to flag any irregularities that suggest AI processes are operating outside their
designed parameters.
By adopting these practices, and with the audit committee’s endorsement of a forward-looking stance, organisations can position themselves not only to confront existing AI risks, but also to anticipate and adapt to future challenges in AI governance. This approach ensures that the organisation is equipped to harness AI’s benefits, while safeguarding against its potential pitfalls.
Patrick Ladon is Vice-President in Group Audit at Deutsche Bank.
This article was published in March 2024.