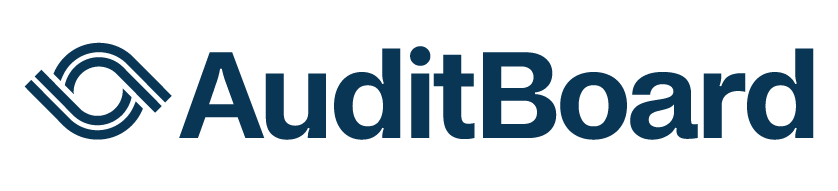
Sponsored Content
4 best practices for deploying and optimising AI in internal audit
By Vi Tran, AuditBoard Product Marketing Manager
Artificial intelligence (AI) will transform how internal auditors do their work. This much is clear. Many internal auditors, however, are still unclear on how AI is being applied in real-world settings. Facing uncharted territory, they’re unsure how and where to begin.
Fortunately, many internal auditors have already met considerable success in deploying AI — and they’re willing and eager to share all they’ve learnt. I was privileged to lead a lively discussion with two risk and controls experts with extensive experience implementing AI in their organisations. As Managing Director in Audit for Models, Data, and AI at Lloyds Banking Group, Shehryar Humayun brings experience as both an audit leader and software engineer. Kunal Pradhan, an IT audit specialist experienced in upskilling teams in new technologies, heads AI in SOX compliance for technology innovator and compute platform Arm.
Sharing their passion for data, automation, innovation, and collaboration, Shehryar and Kunal delivered an inspiring discussion filled with lightbulb moments and pragmatic advice. In particular, they shared four AI implementation best practices sure to help any internal audit team get on the path to effectively deploying and optimising AI.
1. AI shouldn’t be a solution in search of a problem
Setting out, it can be tempting to adopt the AI itself as the starting point: first selecting an AI technology, and only then exploring what problems it might solve. That approach can lead to automating for the sake of automating — not because there’s a proven, cost-effective need. Kunal said, “The one thing I keep reiterating to my team is that AI should not be a solution trying to find a problem.”
Instead, identify problems worth solving by empowering your team to share their day-to-day challenges. Kunal cautioned, “There can be an element of nervousness, with people thinking that because they don’t know how to solve a problem, they’re not going to raise their hand. The culture I’m trying to inculcate within my team and in general is that it doesn’t matter if you know how to create the solution. Tell us what your issues are and how we can help you save time in the audit world. Then, together with our team, engineers, and third parties, we’ll try to find a solution and see if it’s worth spending the time and money.”
Kunal and Shehryar shared several use cases, including:
- Validating issue categorisation, driving root-cause insights, and improving remediation by applying supervised machine learning to the issue management process.
- Gaining a broad view on internal audit’s work and key themes by transcripting walkthroughs, uploading to a SharePoint database, and querying with a CoPilot agent.
- Building a foundation for risk and controls matrix (RACM) work by feeding transcripts into Miro AI to create a first-draft process flow chart.
- Identifying key attributes not captured in walkthroughs by feeding transcripts and a masked RACM to ChatGPT and engineering prompts to query it.
2. Cultivate a culture of effective AI experimentation
Many teams find they lack the right skill sets to use AI effectively, making proactive training essential. Shehryar highlighted four key areas.
- Identifying use cases: Shehryar emphasised the critical importance of “upskilling auditors in the art of the possible” to help them recognise relevant use cases. Creative, innovative thinking is vital for maximising AI’s potential.
- Engaging with AI: Teams must appreciate the necessity of the “human in the loop,” ensuring application of appropriate judgment and skepticism to AI models and outputs. Learning effective engagement is also vital for helping practitioners envisage their future in the age of AI. As Shehryar put it, “Let the machine do the ordinary, so the auditors can do the extraordinary.”
- AI process awareness: AI models involve significant time, effort, and complexity. Shehryar explained, “Auditors need to be aware of not only the requirements they need to have, but also the time it takes to get the right data and the different systems they need to access.”
- AI explainability: Only explainable AI should be used. As Shehryar says, “We need to be able to justify the samples we’ve taken as well as the outcomes we’ve come to.” Some libraries give the reasoning behind outcomes (e.g., basis for identifying outliers).
To support these objectives, training efforts in Shehryar’s team include:
- Prompt engineering sessions. Because prompt engineering can be quite specific to the AI tool and use case, Shehryar holds customised sessions. Coursera, The Institute of Internal Auditors (IIA), and other organisations also offer training.
- Sessions sharing current AI use cases to inspire day-to-day use.
- AI standard operating procedures (e.g., when to engage the analytics team ahead of audits).
- An app store with proven AI tools already in use in the organisation.
3. Collaborate across functions to expedite impact
In most organisations, multiple functions are simultaneously experimenting with AI — and often trying to solve similar problems. Proactively collaborating to share approaches and ideas can be a brilliant way to expedite AI solution creation and impact.
This was a lightbulb moment for Kunal: Like him, many of Arm’s other departmental leaders were learning prompt engineering and developing bots. “Everyone was working in silos and creating these really cool things. Arm has since broken down the silos and gotten departments’ AI champions communicating.” Kunal explained, “What we have realised is that a lot of the problem statements are aligned amongst different teams. And they’ve already found a solution.” For example, the CoPilot agent Kunal’s team created to query transcripts in SharePoint was originally built by Arm’s legal team to check contracts.
4. Get started ASAP and learn as you go
Lastly, don’t let inexperience or uncertainty prevent progress. Kunal advised “the sooner the better, rather than waiting to find the right way to do it. You don't have to have it right in the first instance.” It’s better to get a foot in the door, begin learning what works and what doesn’t, bring in the AI governance team and AI champions as needed, and keep iterating.
The only certain way to fail with AI is never to begin. Fortunately, as our panelists counselled, the path is becoming clearer: Identify the problems worth solving, educate your team, collaborate across the organisation, and start experimenting today.
About the author
Vi Tran, CPA, CIA, is a Product Marketing Manager at AuditBoard, where she leverages her background in audit and internal controls to enhance marketing strategies and outreach efforts. Prior to joining AuditBoard, Vi served as an external auditor at PwC, Internal Controls Senior Analyst at Targa Resources, and Associate Manager at The Siegfried Group.